How to properly train artificial intelligence.
If correctly programmed, artificial intelligence can relieve a lot of human workload. But things do go wrong whenever it has been trained using the wrong data. So how does AI learn to do the right thing?
- If you train a self-learning algorithm using the wrong data there’s a risk of mishaps.
- “Only clean data will prevent machines from making the wrong decisions.”
- Measurable successes at EOS: incoming payments raise up eleven percent thanks to the use of AI.
The “hotness” filter in photo-editing app 'Faceapp' shows what happens when a self-learning algorithm is trained using the wrong data. Two years ago, photos of dark-skinned people were suddenly lightened to make them whiter. The reason for the change in skin color was that the AI had been trained using only one dataset containing light-skinned Caucasian faces. If the AI training had taken account of all ethnic groups this mishap would not have occurred.
Disadvantaged by poor data.
Someone who knows how to correctly train AI systems is Andreas Dix from the Data Science Team at EOS in Germany. The data specialist trains machines for repetitive and time-consuming processes. “Only clean data prevents machines from making wrong decisions.”
“We need to know exactly where the relationships are so that artificial intelligence works properly based on our training”
One way to avoid these mishaps is through proper data exploration. That means approaching the dataset without hypotheses, i.e. impartially and without unconfirmed assumptions. Afterwards, the expert tries to find out what kind of usable information the dataset contains. Are there variables in it that do not have any dispersion? Or does it include variables that have too many missing values? These data should be excluded because they can have an incorrect influence. “We need to know exactly where the relationships are so that artificial intelligence works properly based on our training,” says Dix.
Intelligent programming.
The machine learning algorithms need clean data to recognize structures and draw conclusions. “The rules and conditions set up by the algorithms during the training must not be too specific, because they then will have no value at all for really predicting something. This is then called overadaptation. It would be better to generalize, i.e. find fewer specific structures and as a result achieve good accuracy, with newly acquired data as well.” This can be achieved, for example, by optimizing the hyperparameters of the algorithm and through more training data.
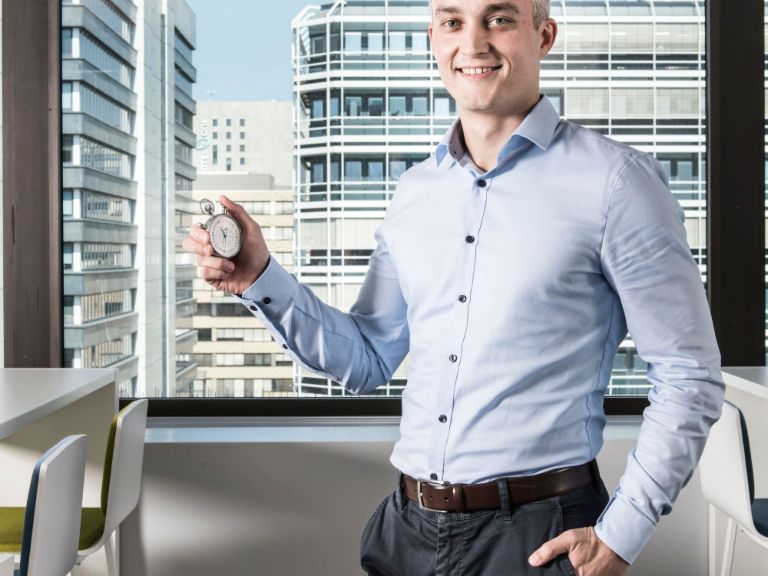
Fully automated receivables.
In relation to debt collecting activities at EOS, this means for example that AI can predict the best collection step to be taken next. Specifically, the data existing in the system up to this point about the receivable itself and the defaulting payers are collected, aggregated and prepared. Only then are all models queried with this data, to predict how successful each collection activity will be for this particular receivable at this point in time. Or to put it more clearly: how much payment inflow can EOS expect. Finally, the activity that is rated as the best option after applying all criteria will be executed by the debt collecting system.
Measurable successes.
Measurable successes have already been recorded thanks to the use of AI at EOS. “At EOS in Germany we have been making productive use of the data-driven AI system D3, Data Driven Decisions. We use it to control the collection process with the result that payment receipts are up around 10 percent. This means that we are achieving five percent higher earnings after activity costs are deducted compared with our previous receivables processing system,” says Dix.